Understanding Machine Learning: A Comprehensive Brief
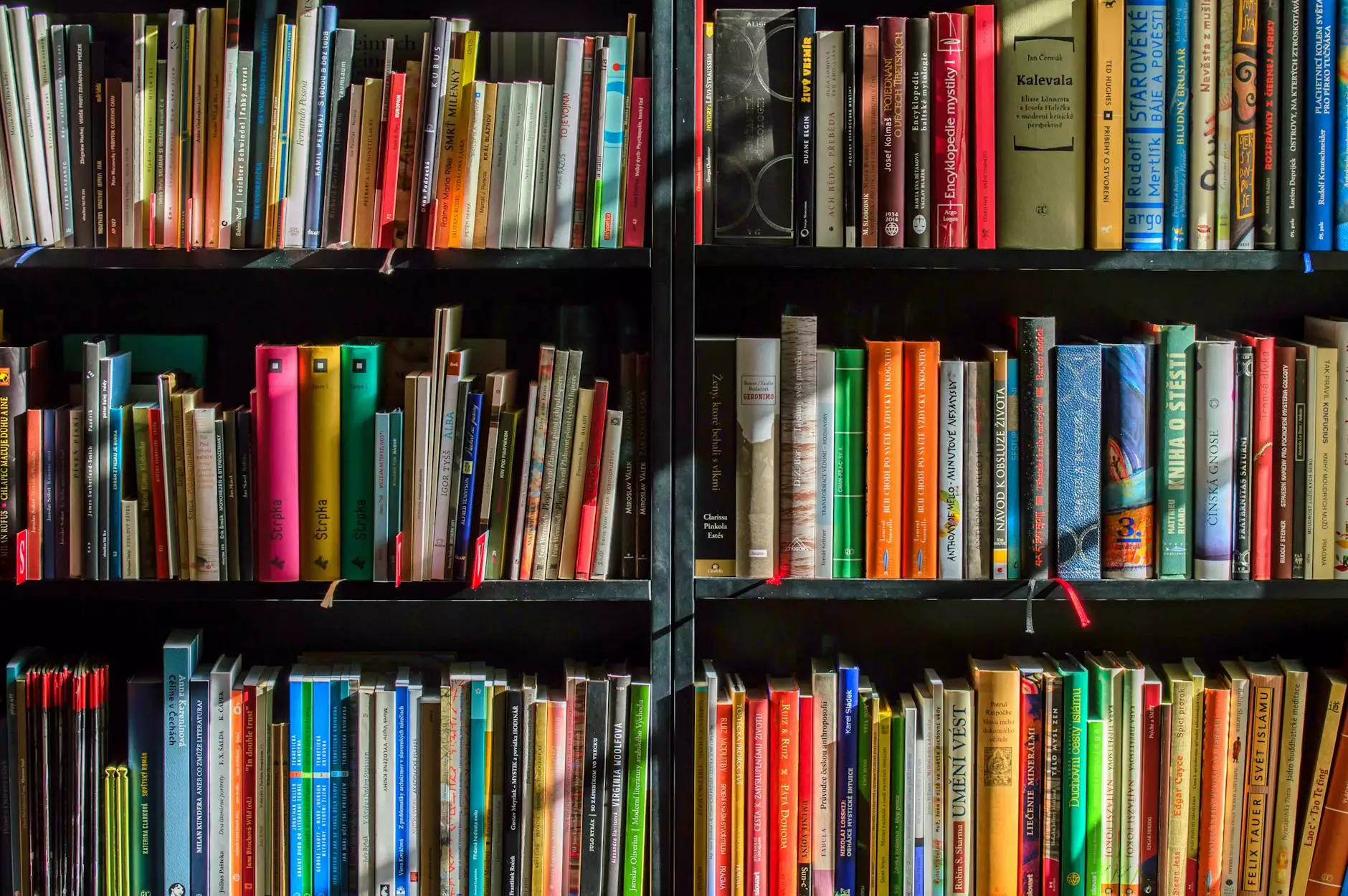
Machine learning has emerged as a fundamental breakthrough in the field of technology, enabling computers and systems to learn from data and improve their performance without explicit programming. In this comprehensive brief about machine learning, we will explore its definitions, types, applications, and the profound impact it is having on businesses across the globe.
1. What is Machine Learning?
Machine learning is a subset of artificial intelligence (AI) that focuses on the development of algorithms that enable computers to learn from and make predictions based on data. Unlike traditional software programming, where a developer manually codes instructions, machine learning systems utilize data to discover patterns and build models that can be used for decision-making.
1.1 The Evolution of Machine Learning
The journey of machine learning began in the 1950s, but significant advancements were made in the 1990s and 2000s with the advent of more powerful computing technologies and the explosion of data. Today, machine learning is fueled by vast datasets and powerful algorithms, allowing it to tackle problems previously deemed insurmountable.
2. Types of Machine Learning
Machine learning can be classified into three main categories:
- Supervised Learning: This approach involves training a model on a labeled dataset, meaning the algorithm learns from examples with known outcomes. Common applications include classification and regression tasks.
- Unsupervised Learning: Here, the algorithm is provided with data that has no labels and must find hidden patterns or intrinsic structures. Techniques include clustering and association.
- Reinforcement Learning: This type of learning occurs when an agent interacts with its environment, receiving rewards or penalties based on its actions. It is often used in robotics, gaming, and navigation.
3. Machine Learning Applications
Machine learning is transforming multiple industries by offering innovative solutions and improving efficiencies. Here are some notable applications:
3.1 Healthcare
In the healthcare sector, machine learning algorithms can analyze medical data to predict patient outcomes, personalize treatment plans, and enhance diagnostic accuracy. For instance, predictive analytics can help in identifying individuals at risk of developing certain diseases based on their lifestyle and genetic factors.
3.2 Finance
The finance industry leverages machine learning for fraud detection, algorithmic trading, and credit scoring. By analyzing transaction patterns, machine learning systems can flag unusual behavior and alert institutions to potential fraud, thereby protecting both the company and its customers.
3.3 Retail
In retail, machine learning enables personalized shopping experiences through recommendation systems that analyze customer behavior and preferences. Retailers can also optimize inventory management, forecasting, and pricing strategies, leading to improved bottom lines.
3.4 Manufacturing
Machine learning helps manufacturers by predicting machinery failures and optimizing supply chain operations. By utilizing predictive maintenance, companies can avoid costly downtimes and enhance operational efficiencies.
4. Benefits of Machine Learning for Businesses
The integration of machine learning into business operations offers numerous benefits:
- Enhanced Decision Making: With advanced data analytics, businesses can make more informed decisions backed by empirical evidence.
- Cost Efficiency: Automation of processes through machine learning reduces labor costs and increases productivity.
- Improved Customer Experience: Personalization through machine learning leads to greater customer satisfaction and loyalty.
- Innovation: Machine learning fosters innovation by enabling new products and services that were not previously possible.
5. Challenges in Machine Learning Implementation
Despite its benefits, the implementation of machine learning does come with challenges:
- Data Quality: High-quality, relevant data is critical for effective machine learning. Poor data can lead to inaccurate models.
- Algorithm Selection: Choosing the right algorithm for the specific problem at hand requires deep understanding and expertise.
- Resource Intensive: Machine learning applications demand significant computational resources, which can be a barrier for small businesses.
- Ethical Considerations: Issues such as bias in algorithms and data privacy must be addressed to ensure ethical AI practices.
6. The Future of Machine Learning
The future of machine learning is bright and promising. With continuous advancements in computing power and algorithm development, machine learning is poised to become even more integrated into everyday life. Here are some predictions for the future:
- Increased Automation: More industries will adopt machine learning for automating repetitive tasks, freeing up human resources for strategic decision-making.
- AI in Creative Processes: Machine learning will penetrate creative industries, assisting in music composition, art generation, and content creation.
- Greater Interoperability: Machine learning systems will increasingly work together in a unified AI framework, leading to more seamless interactions between technologies.
- Regulatory Frameworks: As machine learning becomes more prevalent, regulatory bodies will likely develop guidelines to ensure responsible use and protect consumers.
7. Getting Started with Machine Learning
For businesses looking to harness the power of machine learning, taking the first steps can be daunting. Here is a roadmap to guide the implementation:
7.1 Define Your Objectives
Clearly outlining what you want to achieve with machine learning is crucial. Whether it's enhancing customer service, improving sales forecasting, or automating processes, understanding your goals will guide your efforts.
7.2 Data Collection and Preparation
Gather relevant data needed for training machine learning models. Ensure that data is clean, well-organized, and sufficient to model the scenarios you're targeting.
7.3 Choose the Right Tools and Frameworks
Utilize machine learning platforms and libraries such as TensorFlow, PyTorch, or Scikit-learn, depending on your team's expertise and project requirements.
7.4 Test and Iterate
Implement iterative testing to refine models and improve accuracy based on feedback and performance metrics.
7.5 Scale Up
Once the model demonstrates success, consider scaling it up to impact larger segments of your business.
8. Conclusion
In conclusion, this brief about machine learning highlights the transformative potential of this technology across various domains. As businesses increasingly adopt machine learning solutions, they unlock new opportunities for efficiency, innovation, and growth. By understanding the principles, applications, and challenges of machine learning, organizations can pave the way for a future where AI-driven decision-making becomes the norm.
Investing in machine learning does not merely equip a business with new tools; it establishes a roadmap towards adopting a culture of innovation and responsiveness in a rapidly changing digital landscape.