Creating High-Quality Image Datasets for Object Detection
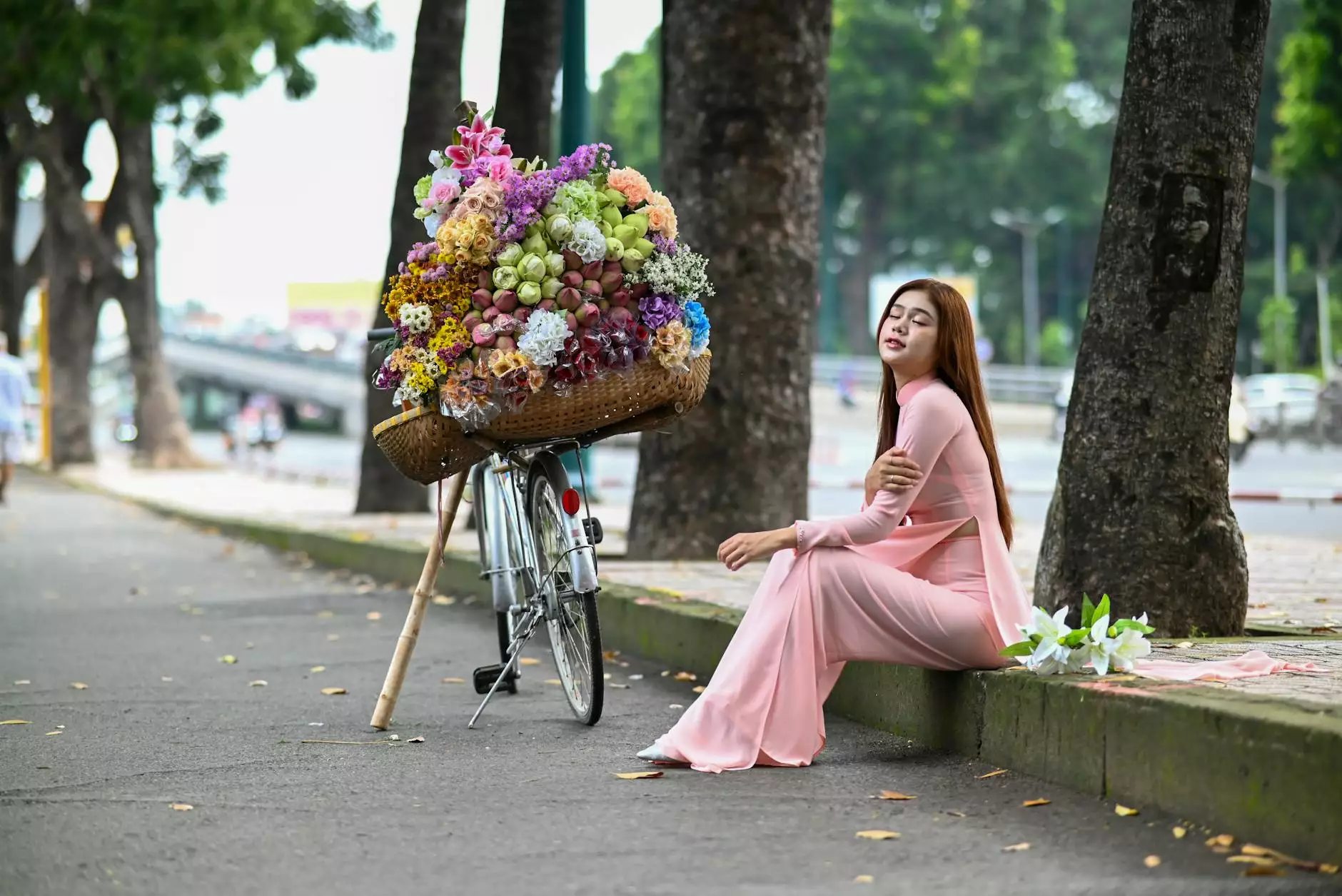
In the rapidly evolving field of artificial intelligence and machine learning, one of the foundational elements for developing effective object detection systems is the utilization of image datasets. An image dataset for object detection consists of a large collection of images that are meticulously labeled to allow automated systems to recognize and identify objects. Ensuring that these datasets are well-curated and properly annotated is crucial for the accuracy and performance of machine learning models.
The Significance of Image Datasets in Object Detection
Object detection is a critical function in various applications such as autonomous vehicles, security systems, and even augmented reality. The performance of these applications is directly linked to the quality of the datasets used to train them. Therefore, understanding how to create and manage an image dataset for object detection cannot be overstated.
Understanding Object Detection
Before diving into the intricacies of dataset creation, let's clarify what object detection entails. Object detection is the task of identifying and localizing objects within images or videos. This process consists of two main components:
- Classification: Determining what objects are present in an image.
- Localization: Identifying where in the image these objects are located, typically using bounding boxes.
Why Quality Matters: The Role of Annotation
The quality of an image dataset for object detection hinges significantly on how well the images are annotated. Proper annotation involves labeling images with precise and accurate information about the objects present. Here’s why quality matters:
Impact on Machine Learning Models
The performance of machine learning models is heavily influenced by the training data they are given. Poorly annotated data can lead to:
- Inaccurate models that fail to detect objects.
- Increased error rates and reduced efficiency.
- Models that can’t generalize well to new, unseen data.
To mitigate these risks, we recommend using reliable data annotation tools and platforms such as KeyLabs.AI to ensure that your annotations are both precise and consistent.
Steps to Create a High-Quality Image Dataset
Creating a high-quality image dataset for object detection involves several critical steps:
1. Define Your Objectives
Before you begin gathering images, it’s essential to clearly define the objectives of your dataset. Ask yourself:
- What kind of objects do I want to detect?
- What environments will these objects be captured in?
- What range of variations in appearance and orientation do I expect?
2. Gather Diverse Images
Diversity is key when collecting images. Your dataset should include images of objects in different conditions, orientations, and backgrounds. This diversity will help your model to learn effectively. Consider using:
- Images from different sources, such as public domain image repositories or your own photography.
- Data augmentation techniques to artificially enhance your dataset and prevent overfitting.
3. Utilize Effective Annotation Tools
Once the images are collected, the next step is annotation. Using effective annotation tools like those offered by KeyLabs.AI can simplify and enhance this process. Some popular annotation types include:
- Bounding Boxes: Draw boxes around objects of interest.
- Polygon Segmentation: More precise outlines of complex shapes.
- Keypoint Annotation: Specific points of interest on objects.
4. Review and Validate Annotations
After the initial annotation phase, it is crucial to have a review and validation step. This ensures that:
- Annotations are accurate and match the intended object.
- Inconsistencies or errors are identified and rectified.
- Overall dataset quality is maintained to ensure reliable model training.
Best Practices for Managing Image Datasets
Once created, your image dataset for object detection needs effective management. Here are some best practices:
1. Organize Your Data Efficiently
Invest time in organizing your data files. A clear folder structure can greatly enhance usability. For example:
- Create separate folders for different object categories.
- Use a consistent naming convention to easily find files.
2. Version Control
Keeping track of changes in your dataset over time is vital. Implementing a version control system can help you:
- Maintain different versions of datasets for various experiments.
- Rollback changes if new annotations cause issues.
3. Continuous Improvement
The process of dataset creation is iterative. Continued feedback and improvement should be pursued:
- Regularly update your dataset with new images and annotations.
- Evaluate the performance of models and use those insights to refine your dataset.
The Business Edge: Leveraging KeyLabs.AI for Data Annotation
To excel in today’s competitive market, leveraging data annotation platforms like KeyLabs.AI is not just beneficial, it’s essential. Here’s how they can help your business:
1. Automation of Annotation Processes
Advanced data annotation platforms automate many tedious aspects of the annotation process, significantly reducing the time spent on manual annotation and increasing efficiency.
2. Scalability
When your needs grow, so can your data annotation capabilities. KeyLabs.AI can scale up to handle larger datasets as your business evolves.
3. Enhanced Accuracy
With High Precision Quality Control systems in place, your image dataset for object detection will maintain high accuracy levels that are critical for effective model training.
4. Cost Efficiency
Leveraging a comprehensive platform can save you both time and costs associated with data collection and annotation processes.
Conclusion
In summary, the importance of a well-prepared image dataset for object detection cannot be understated. From careful planning and diverse image collection, to utilizing cutting-edge annotation tools and the best practices for management, each step contributes to building a dataset that will provide value for your AI projects. By leveraging platforms like KeyLabs.AI, businesses not only ensure efficiency but also gain a competitive advantage in the data-driven landscape of tomorrow.
Invest in your future by enhancing your data annotation capabilities and embracing the potential of machine learning today.