Machine Learning Annotation: Harnessing the Power of Data in Business
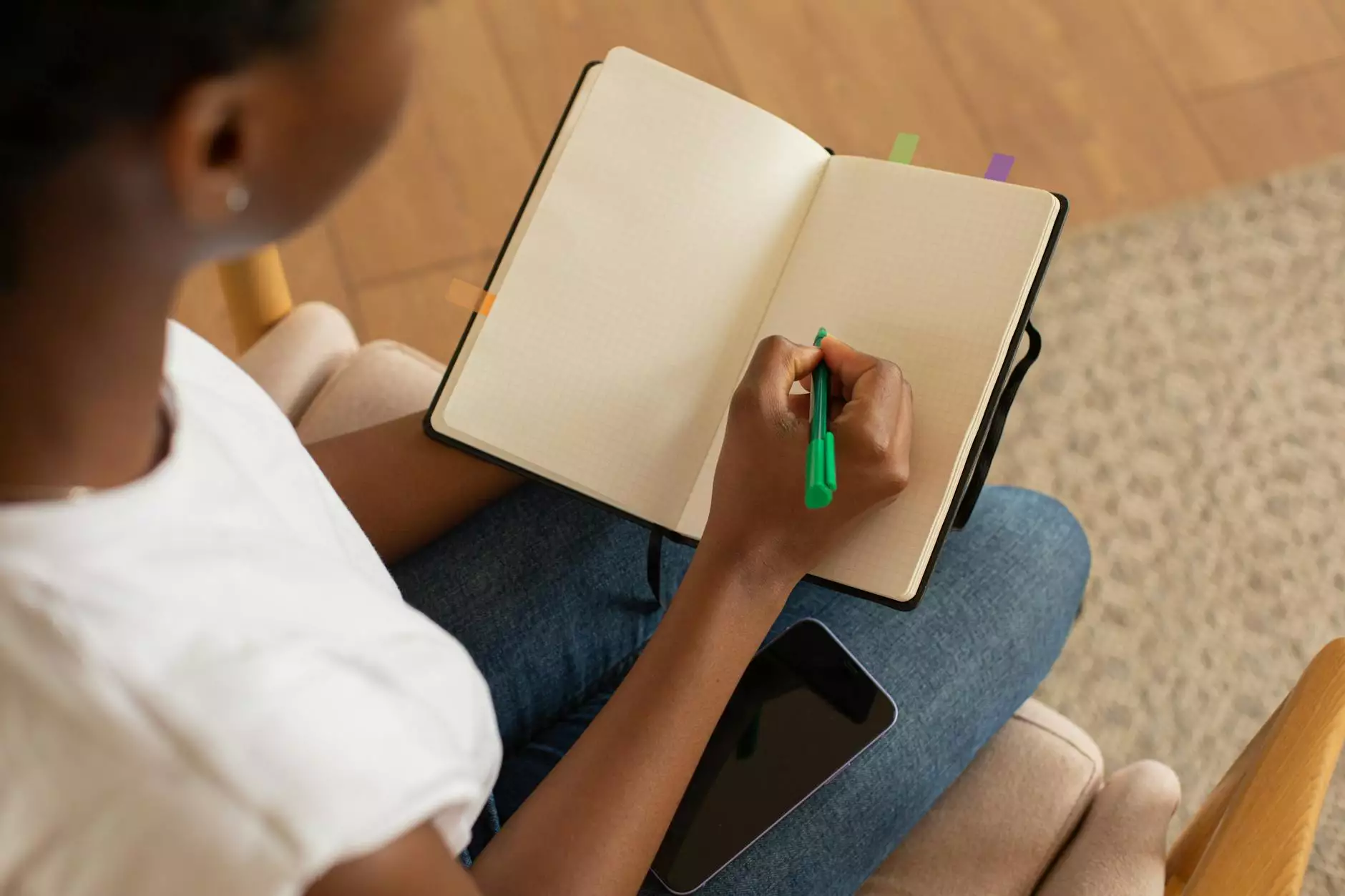
Understanding Machine Learning Annotation
Machine learning annotation refers to the process of labeling data to train machine learning models. It involves various techniques and methodologies to ensure that data is categorized accurately, which is crucial for creating effective AI systems. In today's data-driven world, the significance of machine learning annotation cannot be overstated, particularly for businesses looking to leverage technology for competitive advantage.
The Role of Machine Learning in Business Operations
As businesses across various sectors, including Home Services and Keys & Locksmiths, increasingly rely on technology to enhance efficiency and customer satisfaction, machine learning plays a pivotal role. Here’s how:
- Data-Driven Decision Making: Machine learning helps businesses analyze large datasets to uncover patterns and trends, enabling informed decisions.
- Enhanced Customer Experiences: By utilizing machine learning annotation, companies can personalize services and communications, improving customer satisfaction.
- Operational Efficiency: Automation of routine tasks through machine learning leads to significant efficiency gains, allowing organizations to focus on value-added activities.
Why Annotation is Crucial for Machine Learning Models
The effectiveness of machine learning models is largely dependent on the quality of the input data. Annotation is essential because:
- Quality Assurance: Well-annotated data ensures that the models are trained on accurate information, resulting in better predictive performance.
- Contextual Relevance: Annotation provides context to the data, helping algorithms understand the significance of different inputs.
- Reduction in Errors: Properly annotated datasets reduce the likelihood of errors during model training, leading to improved accuracy.
Types of Machine Learning Annotation
There are various types of machine learning annotation, each suited for different applications. Below are some common types:
- Image Annotation: Labeling images for computer vision tasks. Examples include bounding boxes, segmentation, and keypoint annotation.
- Text Annotation: Involves tagging text data for natural language processing (NLP) tasks, such as sentiment analysis and named entity recognition.
- Video Annotation: Labeling video data for dynamic scene analysis. This can include tracking objects or scene segmentation.
The Impact of Machine Learning Annotation on Home Services
In the Home Services industry, effective utilization of machine learning annotation can lead to transformative results, such as:
- Predictive Maintenance: Annotated data can help develop models that predict when equipment will fail, allowing businesses to perform maintenance before issues arise.
- Smart Scheduling: Machine learning can analyze data on service requests to optimize scheduling, leading to reduced wait times for customers.
- Improved Marketing Strategies: By understanding customer behavior through annotated data, businesses can tailor marketing efforts to target specific demographics effectively.
Advantages of Machine Learning Annotation for Locksmith Services
The application of machine learning annotation in the Keys & Locksmiths sector provides various advantages:
- Enhanced Security Solutions: Annotated data can help develop more sophisticated security solutions by analyzing patterns in theft and break-in data.
- Customized Customer Solutions: By understanding client needs better through data analysis, locksmiths can offer more personalized services.
- Automated Customer Service: Implementing chatbots powered by machine learning can improve customer service availability and responsiveness.
Challenges in Machine Learning Annotation
While the benefits of machine learning annotation are evident, several challenges can hinder its implementation:
- Data Privacy Concerns: Businesses must navigate regulatory challenges regarding data privacy when annotating sensitive data.
- Resource Intensive: Annotation can be time-consuming and require significant human and technological resources.
- Quality Control: Ensuring consistent quality across large volumes of annotated data can be challenging.
Implementing Effective Machine Learning Annotation Strategies
To harness the full potential of machine learning annotation, businesses should consider the following strategies:
- Develop Clear Annotation Guidelines: Clearly defined guidelines ensure consistency and quality in the annotation process.
- Utilize Technology: Automated annotation tools can speed up the process and reduce human error.
- Invest in Training: Providing training for annotators is vital to ensure that they understand the nuances of quality data labeling.
The Future of Machine Learning Annotation in Business
The future of machine learning annotation in business looks bright, with advancements in technology promising to enhance processes further. Emerging trends include:
- AI-Assisted Annotation: The use of AI to assist in the annotation process is set to reduce the time and cost involved significantly.
- Collaboration Across Industries: Sectors such as Home Services and Locksmiths may collaborate to develop models and datasets that can benefit multiple fields.
- Real-Time Annotation: As technology develops, the ability to annotate data in real-time will enhance the adaptability of machine learning applications.
Conclusion: Embracing Machine Learning Annotation for Business Growth
In conclusion, machine learning annotation is not just a technical necessity; it is a strategic asset that can drive business growth in various sectors including Home Services and Keys & Locksmiths. By investing in quality annotation processes, businesses can unlock the full potential of their data, improve operational efficiency, enhance customer experiences, and stay ahead in a competitive landscape. Embrace the power of machine learning annotation and watch your business thrive.
© 2023 KeyMakr – All rights reserved.